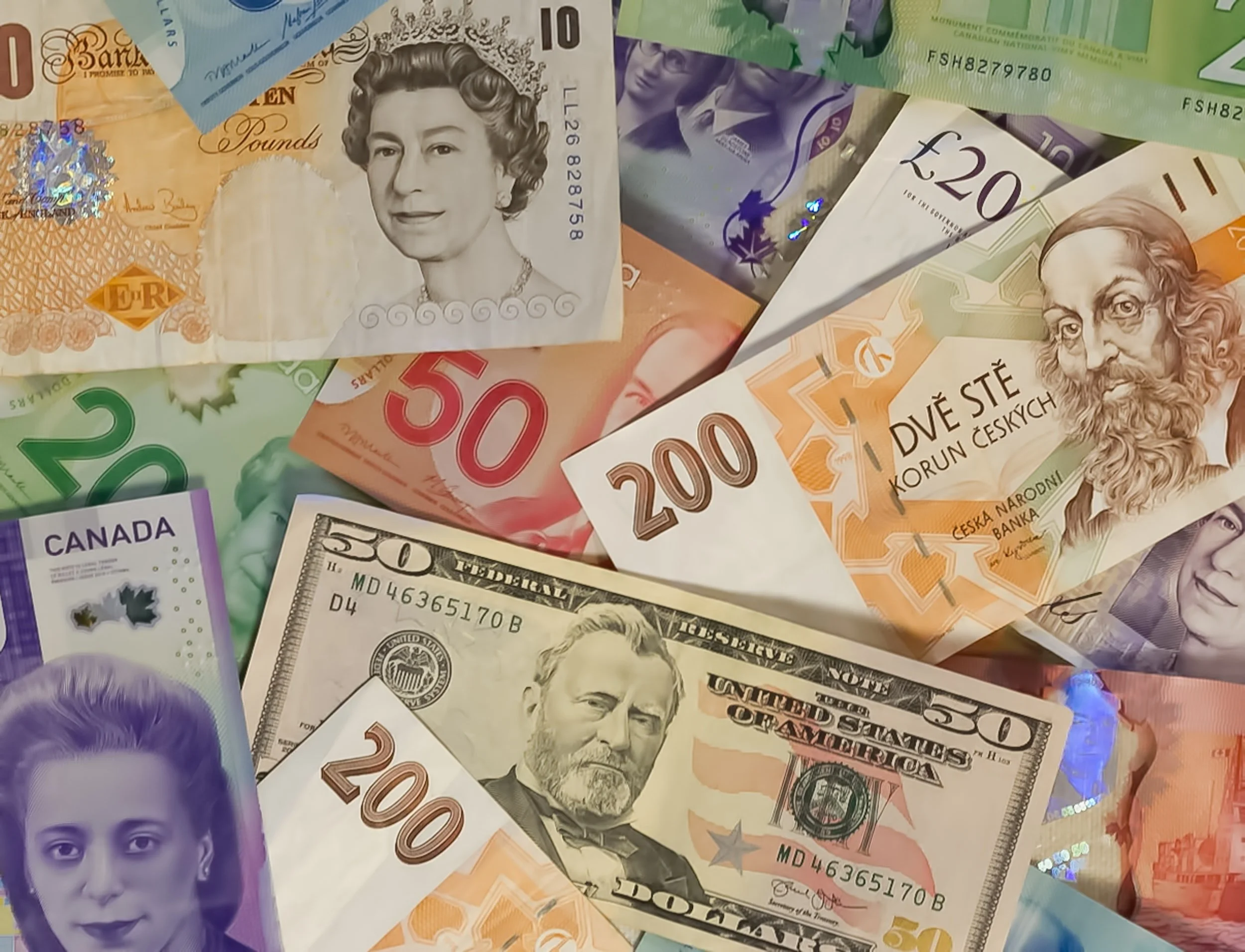
Data Ethics & Applied Analysis for Pig E. Bank
-
Background.
Pig E. Bank, a well-known global bank, is looking for support in its anti-money-laundering department. To achieve this goal, it is looking for a junior analyst to participate in data-related projects to assess client and transaction risk, report on metrics, and build/optimize models to result in a more efficient compliance program.
-
Context.
This project was completed as part of the CareerFoundry's curriculum.
Topics covered were: big data, data ethics, data mining, predictive analysis, time series and forecasting, and using GitHub.
This knowledge was used to uncover leading causes of client loss and identify sources of potential bias, security, privacy, and ethical issues for Pig E. Bank, a fictional global bank.
-
Tools.
Excel - a software application that allows the spreadsheets and their contents to be manipulated.
The Path to Identifying Risk.
Identified sources of potential bias for Pig E. Bank.
Image by Bogomil Mihaylov.
2. Uncovered potential data security, privacy issues, and ethical issues at Pig E. Bank.
3. Cleaned and analyzed Pig E. Bank Customer data in Excel to identify the leading causes of client loss.
Used the insights gained through this data mining for a decision tree.
Table of leading causes of client loss at Pig E. Bank.
Decision tree of client loss assessment at Pig E. Bank.
4. Created a decision tree to determine the probability of customers leaving Pig E. Bank.
5. Evaluated a model for Pig E. Bank.
Image of linear regression model created by CareerFoundry.
Key Findings.
The top five leading causes of client loss at Pig E. Bank are the age, whether the client is an active member, country, gender and balance.
There are some sources of potential bias at Pig E. Bank. Click here for a full list of potential biases.
In some situations, there may be security & privacy issues at Pig E. Bank. Learn more here.
The linear regression model Pig E. Bank created for monitoring fraudulent activity needs to be review further before it is deployed. Please see details here.
Image by Kevin Ku.
Deliverables.
-
Client Loss Risk Assessment
-
Cleaned & Analyzed Data Set
Next Steps.
While the above information fulfills the requirements of the project, the next steps could include:
Work with Pig E. Bank to investigate the potential biases and create a plan to correct any biases that exist.
Work with Pig E. Bank to investigate potential data privacy, data security, and data ethics issues and provide input on how to correct any issues that exist.
Provide support in creating and monitoring a plan to improve customer retention.